5 mistakes that doom your analytics practice to failure
How confident are you that your analytics practice is generating value? The expression 'data is the new oil' is already more cliché than shocking. By now, it's uncommon for organizations to deny the role of data in business. And in response, there are more and more strategic C-Level positions that are in charge of leading analytics use cases (Chief Analytics Officer) and taking care of the organization's data (Chief Data Officer).
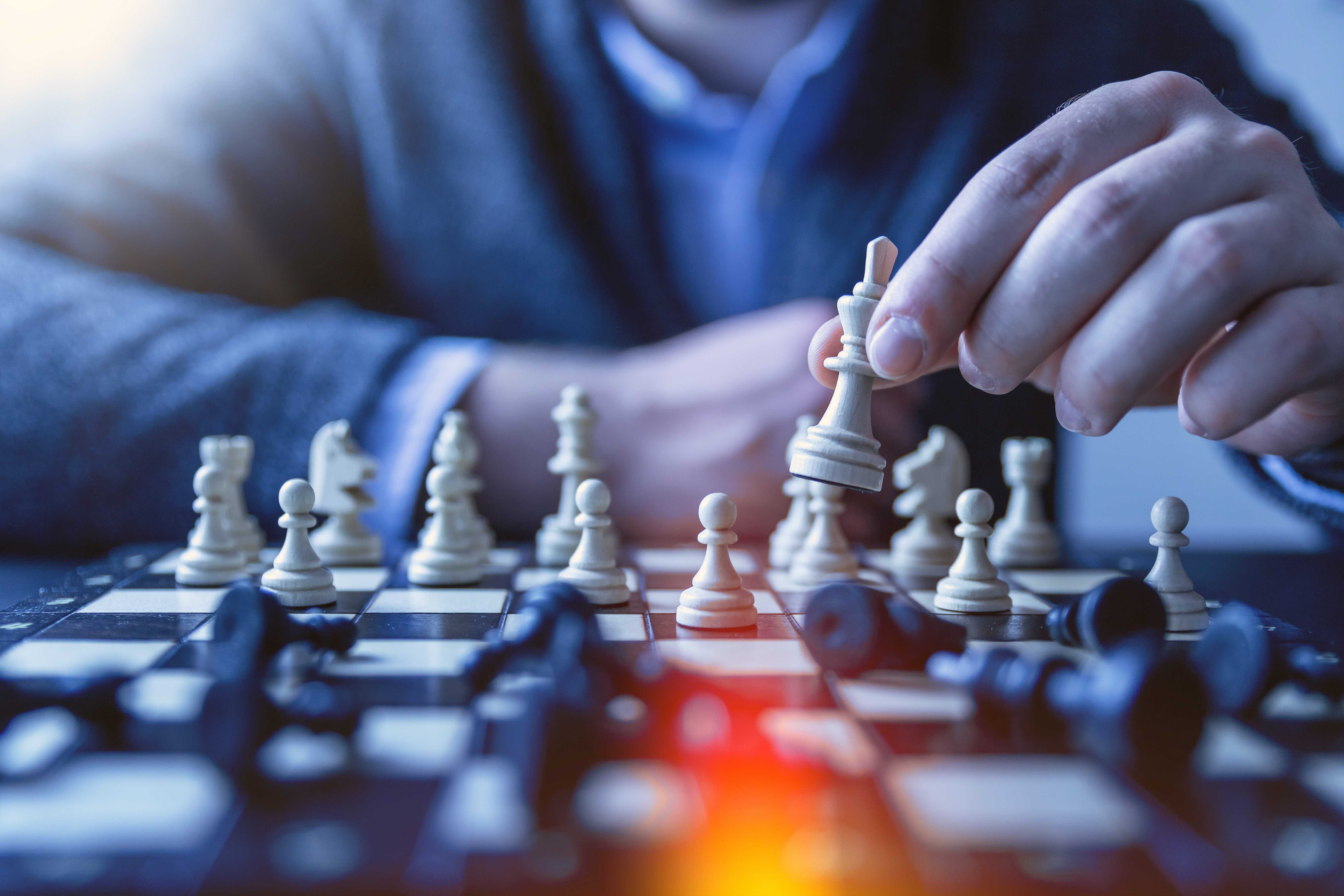
These roles, at all costs, seek to identify optimization opportunities and new business resulting from a joint effort to correctly interpret the signals in data. However, this process of identification and proper execution is more complex in practice than in theory. Many companies have experienced firsthand the consequences of overlooking key issues when establishing a data management and analysis practice.
In this article (part 1 of 2) we will discuss 5 of the 10 most common problems in professionally establishing the practice towards a data-driven culture.
MISTAKE #1: Lack of connection between technical equipment and end-users
In conducting an exploratory analysis, you may see fertile ground for multiple initiatives. Some to improve the customer experience, others to optimize processes
that positively impact quality and reduce waste, and still others to make workers' lives much more bearable... Or at least that's what you think.
It would be irrational to ask a few questions and then just go off and start a project with the team that is now in charge of you. It could be that you have very good ideas. And that you have good developers and designers who will do just what you ask them to do. After all: you are the boss, right? You've even been given a big office and made responsible for analytical innovation (or something like that). You already visualize yourself 3 months from now with a finished product that will hit the bull's eye (those marketing guys don't know what they're in for). Aha. Well, if that's how the novel starts, chances are it will turn and end badly.
One thing you must never forget: if you do not formally involve the experience, opinion and wishes of the key players from the beginning (and their feedback as developments progress) to the extent of making them feel ownership of the initiative: nothing will take off (multiple reasons).
A correct linkage harmonizes technical feasibility (what data do I have?), business knowledge (what rules should I apply?), audience (is it at the right level of complexity/depth?) and end goal (what would a satisfactory deliverable look like?) among other things.
There is an art to balancing these points in order to move forward successfully. A series of sessions under the guidance of a Design Thinking professional is ideal, but ultimately we can say that a brainstorming session, under a framework of respect, with enough intensity and freedom, will be enough to get started.
MISTAKE #2: SETTING EXPECTATIONS TOO HIGH
This point doesn't need much introduction. It is more profitable to be honest with the level of data literacy you have in your company than to throw out the bluff and say that you will implement Artificial Intelligence to give the customer a more personalized experience (After all, you already have two data scientists, right?).
The first thing would be to understand if we have already solved the first link of data analysis: descriptive analytics. That is, if most of my employees can already comfortably talk about historical indicators, metrics and dimensions, in that case, the maturity in the company would be enough to implement something more sophisticated. But, if business users still can't easily get most of the historical analysis you'd like with clean, standardized data in a Dashboard that allows them to visually explore by drill downs, then perhaps the focus should be there first.
Now, if you are already confident that you can explore the grounds of advanced analytics: go ahead, in the end the data will have the last word. What do I mean? Let's say Marketing management wants to send personalized promotions to prospects and customers. Sounds good! Except that until they have a technical session, they discover that the information available is only gender, age, and a history of sales transactions from anonymous buyers. Wow, not a lot of raw material for the team to handle, and you already promised to be able to read signals in the data to identify when a customer would be expecting a baby! Well, new use cases can be explored, and in the worst scenario, a data collection strategy can be initiated.
The summary of this point is: don't promise anything until you have a sense of the data available. Focus on small projects that are feasible with what you have. Once you get going, come up with a strategy to collect better raw material.
MISTAKE #3: Too much (or too little) focus on ROI
Seek balance; yes, that utopian word, which in theory represents a state of perfect harmony between demanding business metrics and the psychological safety of your sales agents, to give an example.
Let's say that in a commercial project with well-defined indicators, you manage to cut contract closing time by 30% and increase the average deal value by 18%. Great, isn't it? Because with that alone, the implementation costs pay for themselves in 3 months. Well, yes, in theory. What you lose
now have to deal with an apathetic sales force that feels watched, burned-out and is looking for other "professional challenges."
Yes, it is important to analyze the financial question in order to move forward with a data strategy. But defining quantitative results so bluntly may blind you to the bigger picture. In the example above, perhaps by not defining the initiative as something to monitor my salespeople and instead to help them be super-powerful negotiators, it will give you results you never anticipated.
MISTAKE # 4: UNDERESTIMATING THE IMPORTANCE OF A METHODOLOGY
Sometimes, the word methodology translates into being slow, bureaucratic and unnecessary documentation that no one will ever read.
The fact is that we agree in part and there is always a lot of controversy around what is the best way to approach "the paperwork" and definitely what is written in the Agile Manifesto is what makes the most sense to us:
Individuals and interactions on processes and tools. Applications that work on very detailed documentation. Collaborative relationships over contractual negotiation.Responding to change over following a plan (set in stone).
This very reasonable way of dealing with digital creations is the most correct from our point of view. However, we do not recommend you to overlook the following documents:
USE CASE. Once you are clear on the objectives, please write them down. Describe the questions you hope to answer, as well as their connection to the strategic business objective. Here, you should record metrics, sponsors, target users, required data, technologies, among other things... This document should be accessible to everyone, including key players' signatures.
KICK OFF MINUTE: Document the project kick-off! There you will be very clear about the elements of the team, the preliminary high-level chronogram, responsible parties and dates.
CLOSURE DISCOVERY. The final product of this phase is usually a detailed prototype, as well as a solution architecture diagram. Many other considerations depending on the maturity of your practice could play here as well, such as: cloud component usage estimates, governance issues, data and user security, among others...
SPRINT PLANNING MINUTES AND CLOSURES. Record the conclusions and if there are any changes or key considerations: make sure they are clear to everyone!
TECHNICAL DOCUMENTATION: The name says it, but the idea is that if John Doe the programmer leaves, no one will ever be able to decipher his logic again. No programmer likes to document, but it is a necessary evil (it is for his own good, ha).
The truth is that this list is an oversimplification of the matter. The point is: don't get so caught up in these documents, maybe no one will ever read them, but what if they do? Remember that when things are done right there is a better chance of success and following a methodology (along with all the paperwork that goes with it) will place you in a flattering position.
ERROR # 5: YOU ARE NOT USING THE LATEST PLATFORM CAPABILITIES
It could be because you do not have the latest versions of your analytical platforms, because there was simply never an upgrade plan from the beginning, or because you do not have the technological arm to continue innovating and strengthening current developments.
Your users, whether internal or external, are already happy (or at least from your point of view), and that's why no one is looking for new things. Come on... are you
in the IT industry not looking to innovate? If you're not keeping up with the latest capabilities, it's your users who will be the judge of your ignorance.
Bring possibilities to the board and evaluate together with them how much value a certain capability would give them and weigh the additional cost to them. For example, ask "if you had that data in real time instead of having it update only at night, what could you do differently?" Or, "imagine if you could define certain thresholds in your data so that every time a certain condition is met an alert is triggered to your cell phone."
Ask, ask, and ask. Don't impose. This is the only way to find out what the business really needs.
Here's the end of Part I. Wait for Part II with 5 additional issues that are dooming your data analytics practice to failure!